
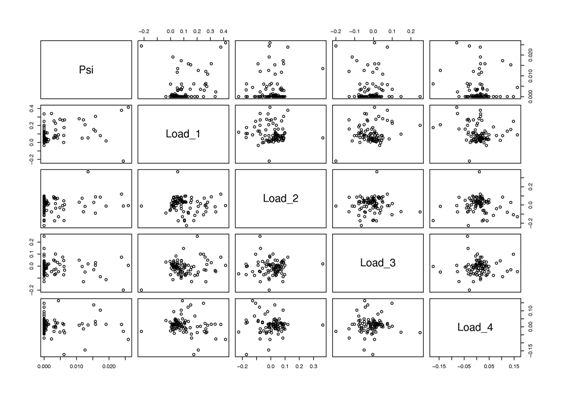
The “experimental” variogram is the plot of observed values, while the “theoretical” or “model” variogram is the distributional model that best fits the data.
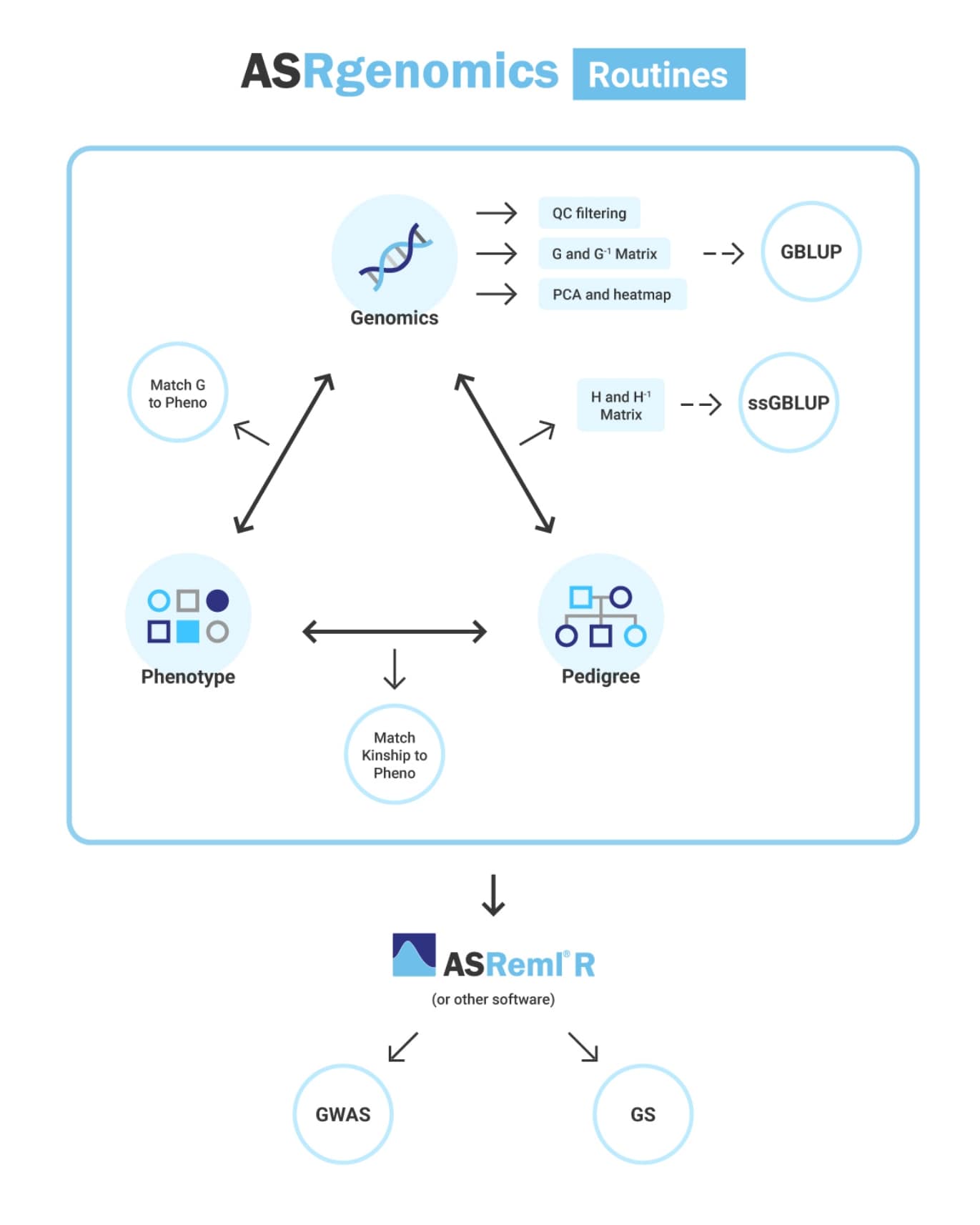
For each pair of points in the sampled data, the gamma-value or “semi-variance” (a measure of the half mean-squared difference between their values) is plotted against the distance, or “lag”, between them. Kriging can be understood as a two-step process: first, the spatial covariance structure of the sampled points is determined by fitting a variogram and second, weights derived from this covariance structure are used to interpolate values for unsampled points or blocks across the spatial field.Ī variogram (sometimes called a “semivariogram”) is a visual depiction of the covariance exhibited between each pair of points in the sampled data. If there is at least moderate spatial autocorrelation, however, kriging can be a helpful method to preserve spatial variability that would be lost using a simpler method (for an example, see Auchincloss 2007, below). Kriging will in general not be more effective than simpler methods of interpolation if there is little spatial autocorrelation among the sampled data points (that is, if the values do not co-vary in space). The value that is generated from the kriging process for any actually sampled location will be equal to the observed value at this point, and all the interpolated values will be the Best Linear Unbiased Predictors (BLUPs). The kriging predictor is an “optimal linear predictor” and an exact interpolator, meaning that each interpolated value is calculated to minimize the prediction error for that point.

This helps to reduce bias in the predictions. Clustering of points is also taken into account, so that clusters of points are weighted less heavily (in effect, they contain less information than single points). In a general sense, the kriging weights are calculated such that points nearby to the location of interest are given more weight than those farther away. Kriging also generates estimates of the uncertainty surrounding each interpolated value. It differs from simpler methods, such as Inverse Distance Weighted Interpolation, Linear Regression, or Gaussian decays in that it uses the spatial correlation between sampled points to interpolate the values in the spatial field: the interpolation is based on the spatial arrangement of the empirical observations, rather than on a presumed model of spatial distribution. An example of a value that varies across a random spatial field might be average monthly ozone concentrations over a city, or the availability of healthy foods across neighborhoods. Kriging is one of several methods that use a limited set of sampled data points to estimate the value of a variable over a continuous spatial field. Kriging is a method of spatial interpolation that originated in the field of mining geology as is named after South African mining engineer Danie Krige.
